References
Convolutional neural networks for wound detection: the role of artificial intelligence in wound care
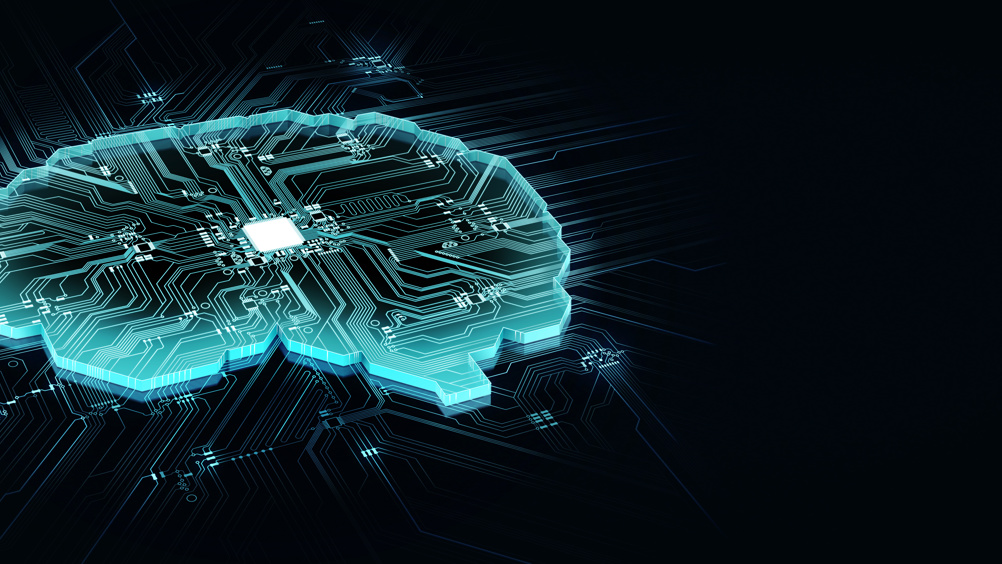
Abstract
Objective:
Telemedicine is an essential support system for clinical settings outside the hospital. Recently, the importance of the model for assessment of telemedicine (MAST) has been emphasised. The development of an eHealth-supported wound assessment system using artificial intelligence is awaited. This study explored whether or not wound segmentation of a diabetic foot ulcer (DFU) and a venous leg ulcer (VLU) by a convolutional neural network (CNN) was possible after being educated using sacral pressure ulcer (PU) data sets, and which CNN architecture was superior at segmentation.
Methods:
CNNs with different algorithms and architectures were prepared. The four architectures were SegNet, LinkNet, U-Net and U-Net with the VGG16 Encoder Pre-Trained on ImageNet (Unet_VGG16). Each CNN learned the supervised data of sacral pressure ulcers (PUs).
Results:
Among the four architectures, the best results were obtained with U-Net. U-Net demonstrated the second-highest accuracy in terms of the area under the curve (0.997) and a high specificity (0.943) and sensitivity (0.993), with the highest values obtained with Unet_VGG16. U-Net was also considered to be the most practical architecture and superior to the others in that the segmentation speed was faster than that of Unet_VGG16.
Conclusion:
The U-Net CNN constructed using appropriately supervised data was capable of segmentation with high accuracy. These findings suggest that eHealth wound assessment using CNNs will be of practical use in the future.
Hard-to-heal wounds include pressure ulcers (PU),1 diabetic foot ulcers (DFU)2,3 and venous leg ulcers (VLU).4,5 The increase in the prevalence of hard-to-heal wounds is an urgent issue that must be resolved in order to relieve pressure on the health-care economy. These wounds are increasingly treated by a range of health professionals in outpatient clinics or in the community, under which circumstances, the use of computer technology and cloud applications using mobile devices, such as smartphones and tablets is becoming more important.
Telemedicine is an essential part of the support system for clinical settings outside the hospital.6 Recently, the importance of the model for assessment of telemedicine (MAST) has been emphasised.7,8 Telecare and telehealth are under a state of rapid development. Of these a fundamental technology is an eHealth-supported wound assessment system using artificial intelligence (AI). However, at present, no device capable of automatically evaluating wounds has been developed, although the segmentation of wounds with considerable accuracy is possible.
Register now to continue reading
Thank you for visiting Journal of Wound Care's Silk Road Supplement and reading some of our peer-reviewed resources for healthcare professionals across Asia. To read more, please register today.